
Real World Data in Healthcare
Here are some datasets I have used over the past 5 years. I think of these data sources as novel simply because they differ from the traditional datasets most economists use (eg: CMS data or national surveys that we are often used to). The goal of this page is for it to be a resource and feel free to reach out if you have questions about any of these datasets

HealthJump - EMR data covering appx 70 million Americans
HealthJump data come from a national platform for managing the collection, storage, and movement of clinical and financial data between EHRs, applications and healthcare organizations. HealthJump connects to providers, and homogenizes their EMR records regardless of their specific EMR vendor. The data includes your typical EMR schemas eg: appointments, procedures, and labs. There are some unique features to these data. We were able to identify when a visit is scheduled, cancelled/missed, which is not possible in claims data. The data is also linked to a provider - so instead of getting a preview from closed claims (what a patient consumes), you get to see a closed provider EMR setup (everything a provider offers to those insured and uninsured). See work here done with these data and work here that describes the data.
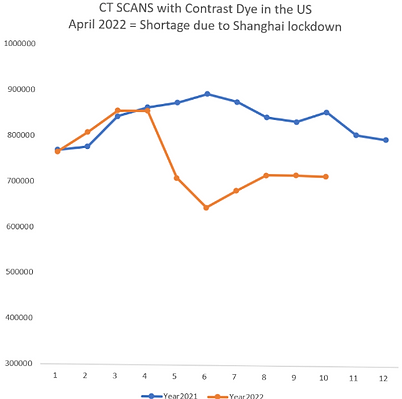
Change Healthcare - A claims clearing warehouse for 170 million Americans
Change Healthcare clears claims for over 170 million insured Americans. The data includes Optum insured individuals along with other insurers. A nice feature is that the data has Medicare Advantage and Medicaid Managed Care patients. Change Healthcare claims include procedures, diagnoses and drug prescriptions. We used these data in 2020 to examine the effect of in-person schooling on Covid19 hospitalizations. We are now using it for various other post Covid ideas.... The data spans 2020 onwards and is updated in real time.
To show you how recent the data is, my co-author Xuechao Qian and I are examining the effects of deferred CT Scans (as a result of the Shanghai shutdown and contrast dye shortage) on patient outcomes. Look at CT scans that need dye in the US in April 2022 when Shanghai shutdown!
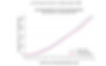
Datavant Death Index Identifiable Obituaries covering 85% of US deaths
Turns out obituaries are a very useful source for identifiable death records. Between 2020 and 2022 we linked almost 85% of all deaths in the US to patients with medical appointments in 2020. We examined the effect of missed healthcare visits due to the sudden onset of the pandemic on downstream mortality. The obituaries we used come from the Common Death Index (an effort on behalf of Datavant and others who use ML techniques to obtain a sizable share of US deaths from web records). The data goes back to the 1800s btw! The chart above shows you the death rate- 12 months later- for ppl with visits scheduled for Feb 2020 vs March 2020. Most of these visits were scheduled well in advance of the pandemic.
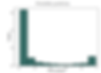
USPTO Patents X NLP models
Data from text is an under utilized resource in economics! Economists have long used patents to examine trends in innovation. This is particularly useful for technologies that do not require clinical trials (eg: medical devices), and so one can not rely on clinicaltrial.gov or the FDA's orange book data. Often patent frequency or citations (eg: forward to backward citations) are studied. Less studied are the textual details of the innovation explained in the patent. Machine learning is changing that with the use of NLP models (see Clemens and Rogers for an example). In our work on dialysis, we used a combination of Word 2 vec and TF-IDF models to characterize traits of innovation in medical devices between 1970 and 2019. Importantly, we wanted to study why one industry moves quicker on one innovation trait (say portability/miniaturization) than another industry. Code for scraping USPTO patent text and running TF-IDF is available to share. We scrapped 80,000 patents, and trained a TF-IDF model to characterize patents based on pre-defined technology traits.

All of Us Research
For a long time I have been envious of the United Kingdom's biobank with genetic data linked to patient records for appx 500,000 Brits. But the US finally got there with the launch of AllofUs. AllofUs aims to enroll 1 million Americans and obtain genetic data, seroprevalence data, EMR records and survey data on them longitudinally. It is also the first real federal attempt at an open EHR system. The data currently (Jan 2023) holds genetic + EMR + survey data for appx 300,000 ppl. It over samples historically minitorized populations - which is great because they are often underrepresented in clinical trial data. The data access is pretty easy. In a soon-to-be-released working paper, we dig into what data AllofUs contains and how the world of genetics and socioeconomics can collide to answer new questions.

Covid19 Common Data Schema
Not everything about the pandemic was bad. One realization that came out of the pandemic was how fragmented US healthcare data is. We are just now attempting to really have open EHR or open Claims data (where we can observe a patient across EHR vendors in EMR data) and maybe one day an all-payer claims dataset for all Americans will exist. The Covid19 Database was an industry initiative that aims to bring data together. The common schema links some big datasets. Eg: You can link mortality data, to web cookies, to EMR records, and to claims. It comes with significant redactions but overall can be useful. See here work by others that linked voter records to mortality, and work by my co-authors and I where we link obituaries to EMR data. Reach out if you need help getting access, or linking datasets using de-identified tokens.